│By Sarah L. Ketchley, Senior Digital Humanities Specialist│
This digital project was prompted by the broad research question: how was archaeology reported in The Illustrated London News (ILN)? The ILN is a publication notable for its fine illustrations and contributions by some of the pre-eminent archaeologists of the day. Gale Primary Sources offers access to the entire run of the newspaper covering the period 1842-2003. This blog post describes a workflow for the preliminary investigation of the data: initial content set creation, cleaning, analysis, export and visualization. At the outset, the research questions were necessarily broad:
- Which words were most prevalent in articles reporting on archaeological digs?
- What themes or topics are most prevalent in the dataset?
- What was the overall feeling about this type of reporting? Was it reported favourably?
- Is it possible to identify which archaeologists were directly contributing to the publication and how many contributions they made?
Engaging in the practical process of curation and analysis offers opportunities to refine these questions, and almost inevitably suggests new avenues for future exploration.
Building my Data Set
I built my data set using the Advanced Search in the Gale Digital Scholar Lab, limiting my search (from what would initially have been the whole Gale Primary Sources corpus) to The Illustrated London News, and for keywords including ‘archaeology’ (which appears for the first time in 1881), ‘excavations’, and ‘ruins’. I also searched by site, excavator and civilization, for example, ‘Layard’, ‘Assyria’, ‘Nineveh’, ‘Sumerian’, ‘Egypt’, ’tomb’ etc. While the returned results certainly weren’t comprehensive, I ended up with an initial content set of 2513 primary source documents, mostly newspaper articles with some advertisements. This corpus comprised a collection of OCR texts generated from original scans of the ILN Historical Archive.
I wanted to examine the content of the documents to see if I could identify recurrent themes or topics, along with the most common words, and expressions of positive or negative sentiment in the dataset. I opted to work with Tableau to generate multiple visualizations for display on an interactive dashboard, and to use Gale Digital Scholar Lab to create the statistical data underlying these visualizations by running a series of text mining analyses using the tools available in the platform.
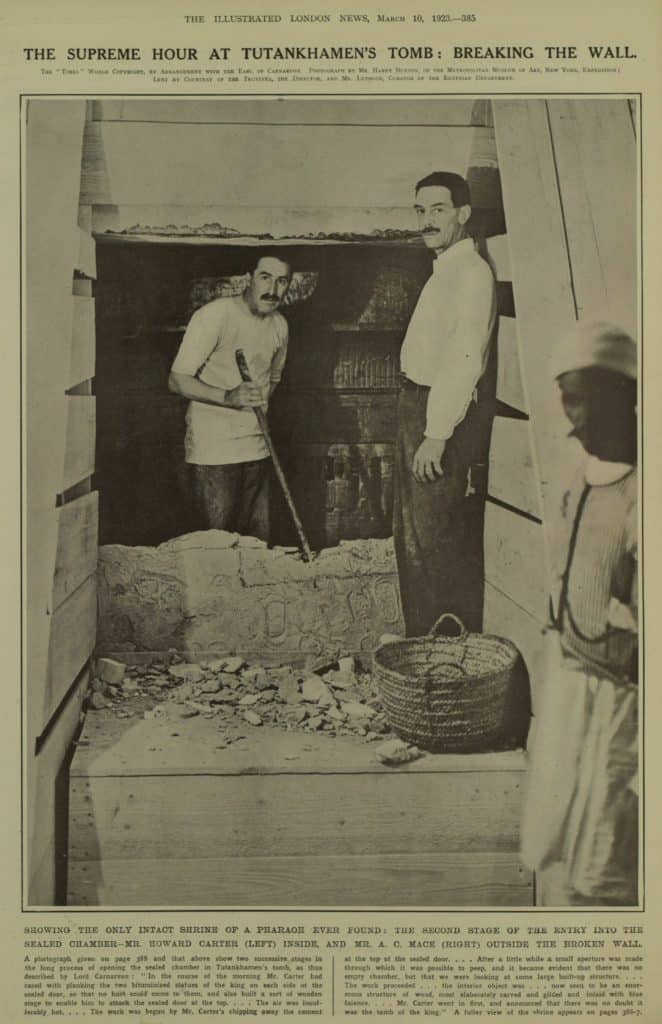
Cleaning the OCR Text
To perform statistical analysis on my content set, I first needed to “clean” the texts to remove recurrent OCR errors. I also removed stop words – the most common words in the English language and not of interest to me for my research. I ran a preliminary nGrams analysis to identify prevalent OCR errors: I configured the tool to return only unigrams (single words) and downloaded the results as a CSV. I was then able to identify and weed out some regularly occurring OCR errors reflected in the CSV, which I pasted into the stop word list in the Clean tool. I continued to iterate on this process, slowly removing and correcting the base OCR texts.
Once I was satisfied that I’d created a clean configuration that removed most of the most common OCR text errors, I ran the collected dataset through my chosen analysis tools.
Using Analysis Tools
- Topic Modelling
Gale Digital Scholar Lab uses the open-source tool named Mallet to perform LDA topic modelling on the corpus of texts. The algorithm iterates through the ‘bag of words’, or collected textual data, and identifies terms which are topically similar, then groups them together. Users can fine-tune the tool configuration – in this case, I chose to run 30 topics each containing 20 topic terms and I applied the cleaning configuration I’d created for this tool. I chose this number of topics because I wanted to move beyond what the algorithm would find most obvious, and instead discern connections that were less apparent in the data set.
I exported the results of this analysis run as a CSV, as well as a second ‘topic proportion by document’ analysis spreadsheet, which I didn’t end up using for this run of visualizations, but which nonetheless provides a wealth of granular detail about my documents. I was able to examine individual articles returned in the results list using the Document Terms output alongside the ‘Documents by Topic’ popup.
- Sentiment Analysis
Gale Digital Scholar Lab visualizes sentiment scores by document, and sentiment across time, using the AFINN sentiment lexicon which ranks documents as positive, neutral or negative on a scale of +5 to -5 based on the words that are included in the text.
I ran this tool on my cleaned dataset and exported the results as a CSV. I anticipated that visualizing this data would give me a sense of how archaeology and archaeological reporting was presented in a popular publication; ideally, I would like to compare this with other contemporary newspaper reporting while also taking in to account authorship – whether the material was written by an archaeologist or by a staff reporter.
- Clustering
This analysis is carried out using the k-means clustering algorithm. I configured the tool to group the documents into 20 clusters according to the algorithm’s ranking of proximity or similarity in document content or other factors, and I again downloaded the CSV output.
I found this output more challenging to visualize in a meaningful way, and ultimately opted to drop clustering in favour of topic modelling, which provided a thematic breakdown sufficiently detailed to be of interest.
Metadata
Finally, I downloaded the metadata for all these documents, which included author, title, date of publication, place of publication and document ID. I wanted to see if I could identify whether archaeologists wrote regularly for the newspaper, and the content and context they provided compared with more formal academic publications. This is where the real challenge began!
Working With the Data in Excel
The date formats in the exported CSV presented post-1900 dates as numbers, and pre-1900 as text. This raised the methodological question of how to clean up these variances, an issue that was compounded by the fact that Excel does not recognize pre-1/1/1900 dates.
I was able to find a couple of useful resources online which ultimately helped me to solve the problem, but I was surprised by how complex it turned out to be.
This article provided good background context, while this post ultimately solved my issue. I began by manually splitting out the text dates into a separate column.
I then created an additional 3 columns to perform the text to number conversion, and to get Excel to render dates before 1-1-1900 appropriately. The process was a success, although one issue that arose was that blank fields were replaced with 1900-01-01, and trying to clean these up with find and replace didn’t work. So again, I had to do this manually, but the final outcome was a table with all dates standardized in the format YYYY-MM-DD.
Using Exported CSV Data in Tableau
- Ngrams
To answer the question ‘which words were most prevalent in articles reporting about archaeological digs?’ I visualized the nGrams CSV output in Tableau. A bar chart proved to be the most effective visualization to clearly demonstrate the output.
Following data clean up, the most common terms included ‘century’, ‘excavations’, and ‘site’, which one would probably expect in documents related to archaeology. The first civilization mentioned is ‘Roman’ and the city is ‘London’ which – given that the newspaper was published in the UK, and Roman archaeological artifacts are regularly found – is perhaps not surprising. Further refinements in the terms may yield interesting results about the civilizations most reported on, and whether the focus was on the material culture or the process of archaeology itself.
- Topic Modelling
I used the circle view in Tableau to visualize my Topic Modelling analysis to answer the question ‘what themes or topics are most prevalent in the dataset?’ I found the option to display ‘all’ or to zoom in on a single theme to be most helpful.
Topic Modelling is a qualitative form of analysis, so it is incumbent on the researcher to decide what the connections are between the terms that the algorithm comes up with and to then name the topic appropriately. In this case, the most common words are grouped around the theme which I named ‘Excavations and finds’. The term displayed above is ‘site’ which occurs 2,323 times in the dataset.
I plan to do more work on this visualization, returning to Gale Digital Scholar Lab in conjunction with Tableau to experiment with various topic measures available in the platform. The granularity of information this form of analysis provides could allow for more detailed results as I continue to refine the OCR content.
- Sentiment Analysis
I used the Sentiment Analysis output to answer the question ‘what was the overall feeling about this type of reporting? Was it reported favourably?’. I imported the CSV and created a sentiment analysis visualization in Tableau using two line charts to show the relative mean sentiment score, and the sentiment score. The data was visualized over time, between 1842 and 2003, which is the full run of the newspaper. Overall, the sentiment is overwhelmingly positive.
Clicking on the ‘most positive’ report brings up the detail of the point, along with the relevant Gale Document ID:
I was then able to go into Gale Digital Scholar Lab to find the document and figure out what made it so positive:
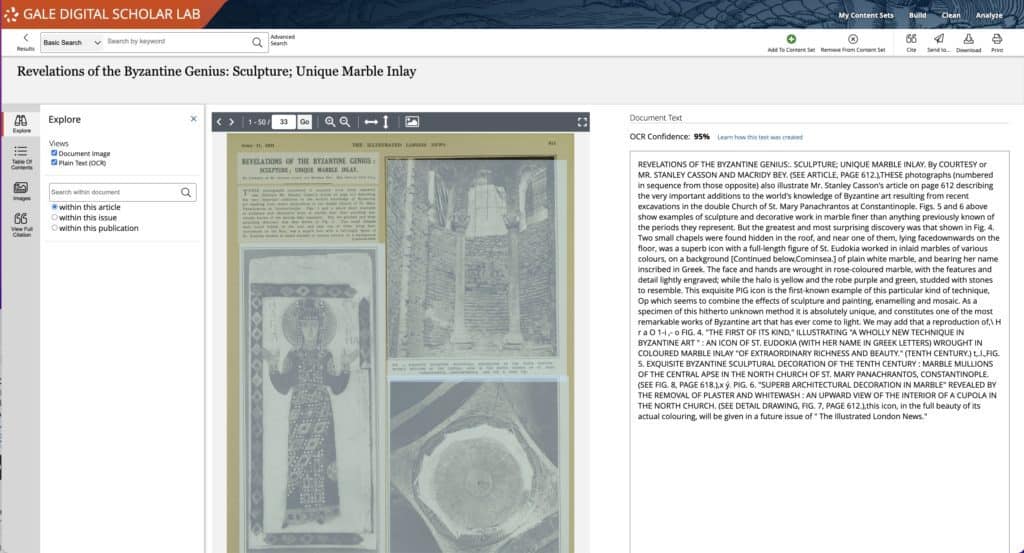
Words like ‘genius’, ‘unique’, ‘important’, and ‘finer’ contribute to the positivity of the document. Looking at the period between the world wars, there was a lot of positive reporting on archaeology, and it was certainly a time of great activity and discovery. I felt that this visualization did a good job of capturing this renewed enthusiasm, while the two line charts gave a good sense of the pervading sentiment in this newspaper.
Archaeologists as Reporters
My final question pertained to the archaeologists themselves: ‘Is it possible to identify which archaeologists were directly contributing to The Illustrated London News and how many contributions they made?’
The answer is yes, but interestingly fewer of the articles prior to 1900 have an author’s name associated with them. I didn’t realize that the articles only started having by-lines after this date until I looked at this visualization. There are several well-known archaeologists authoring articles, including Max Mallowan (who excavated in Iraq and was the second husband of Agatha Christie), Howard Carter/Percy Newberry/Harry Burton/A. Mace (Tutankhamun), Henry Frankfort (early Egypt), J.D.S. Pendlebury (Egypt and Crete), John Garstang (Egypt), and many more. In fact, it was fascinating to see how many archaeologists wrote articles for The Illustrated London News. I plan to compare authorship of similar articles from other contemporary newspapers, for instance, The Times of London, to see if the ILN is an anomaly or the norm.
Tableau Dashboard
My final Tableau Dashboard includes the Ngrams, Sentiment Analysis and Topic Modelling analyses generated by the raw exported data. Ngrams shows that, while the general terms ‘century’, ‘excavation’ and ‘site’ are the most common in my dataset, the most-reported excavations are those with Roman finds. This pattern is reinforced when interacting with the Topic Model: again, the biggest topics include those describing general archaeological terms, while the algorithm also does a good job of grouping by civilization or type of find, including Egyptian, Assyrian, Roman, Greek, Etruscan and pottery, bronze/metal, tomb etc., and giving a snapshot of some of the more significant archaeological activities of the day. Finally, Sentiment Analysis indicates that the period between the two World Wars was the most positive in nature: there were are a large number of excavations under way during this period, with well-reported finds.
While I didn’t include my analysis of authors in the final dashboard, I was able to identify the main contributors to the newspaper, who also happened to be some of the more famous practicing archaeologists of the day. This opened a new research angle to me, as I thought about comparing published excavation reports by these individuals with their more popular writing.
If you enjoyed reading about how archaeology was reported in The Illustrated London News, you may like:
- Nazi Germany, Ancient Rome: The appropriation of classical culture for the formulation of national identity
- Exploring Receptions of Classical Literature with The Times Digital Archive and Gale Digital Scholar Lab
You may also like previous posts in the “Notes from our DH Correspondent” series, which you can find here. Recent posts include:
- Practical Pedagogy with Gale Digital Scholar Lab, Part I: Developing Your Syllabus and Learning Objectives
- Practical Pedagogy with Gale Digital Scholar Lab, Part II: Approaches to Project-Based Teaching and Learning
- A Sense of Déjà vu? Iteration in Digital Humanities Project Building using Gale Digital Scholar Lab