│By Sarah L. Ketchley, Senior Digital Humanities Specialist│
Sentiment Analysis can be described as an exploratory form of analysis that reveals trends or patterns in positive, negative or neutral sentiment of a collected set of documents (a ‘Content Set’). This type of analysis can identify avenues for further research or investigation at both a macro (Content Set) and micro (document) level.1 Sentiment Analysis is one of the six analysis tools available in Gale Digital Scholar Lab where it was recently updated to include an expanded sentiment lexicon which offers opportunities to further refine analysis results. This blog post will discuss these enhancements and offer suggested pathways to work with Sentiment Analysis both in the classroom and for research.
What is the AFINN Sentiment Lexicon?
AFINN is an English word list developed by Finn Årup Nielsen between 2009 and 2011 from tweets related to the United Nation Climate Conference (COP15).2 Words within this list have been ‘scored’ via use of dimensions of emotion/sentiment. The three primary independent dimensions of emotions or sentiment have been defined as:
- valence or pleasure (positiveness–negativeness/pleasure–displeasure)
- arousal (active–passive)
- dominance (dominant–submissive)
It has been argued that individual emotions such as joy, anger, and fear are points in a three-dimensional space of valence, arousal, and dominance.3 Nielsen scored words manually for valence, but left out subjectivity/objectivity, arousal, and dominance. His lexicon is modern in tone, including Urban Dictionary slang and some acronyms; he scores words in a range from -5 (negative sentiment) to +5 (positive sentiment).
The current version of the AFINN lexicon is AFINN-en-165.txt which is also the version now available in Gale Digital Scholar Lab. It contains over 3,300 words with a polarity score associated with each word, and is available in full in Nielsen’s GitHub repository. It can be useful to look through the list as a starting point to gain an understanding of the types of words being scored, and their relative weighting. The list of 3,300 ‘scored’ words includes 905 more than the previous version of the AFINN lexicon; this means sentiment scores in any given text dataset can be more accurately assessed and interpreted by the researcher.
How does Sentiment Analysis work in Gale Digital Scholar Lab?
In the Lab, the Sentiment Analysis (SA) tool is one of six analysis options, all of which are seamlessly linked with both Content Set creation, and the process of cleaning and data curation. The SA tool parses tokens (words or short phrases) from each document and assigns a sentiment score for the document by taking the sum of the scores and dividing it by the number of scored tokens. The Lab’s implementation of Sentiment Analysis is aimed at providing a document-level accounting of affective meaning for documents within a Content Set. At present, it is not designed to analyze sentiment at the sentence or phrase level.
Sentiment is visualized across an overall Content Set or can be mapped over time. Interactivity within the Lab includes the ability to explore individual documents within the visualization, and to remove outlying or irrelevant Content Set material should that be appropriate for the analysis material. The resulting visualization can be exported in various image formats, along with raw tabular data in JSON and CSV formats.
A Sentiment Analysis Case Study: Considering Emotional Responses to the World’s Fair 4
To illustrate the differences between interpreting sentiment though the processes of close and distant reading, I chose to look at reactions to the Exhibition of 1851 represented in contemporary reporting. The starting point was an article written by Cantor in 2015 which selected a range of visitors’ print reports to explore, highlighting the prevalence of the term ‘wonder’ in relation to both architecture and exhibits on display at the Fair.5 Cantor reports overwhelmingly positive feelings conveyed by attendees, whose prose was enthusiastic, amazed, and enchanted. There is some disappointment expressed in the experience of viewing the Koh-i-noor diamond, whose reputation preceded it, as visitors found that the real diamond failed to sparkle inside its burglar-proof case. Cantor’s analysis was carried out by close reading a range of contemporary texts, including newspaper accounts, memoirs, letters, poetry, and catalogues.
He concludes: “Wonder was the word they often used on first sight of Paxton’s otherworldly Crystal Palace. It also seemed the best word in trying to describe the indescribable; visitors not only encountered the recurrent difficulty of expressing emotional experience in language, but the Exhibition was so unique that it was not easily related to previously experienced events and to the existing vocabulary to describe such events. The uniqueness of the Exhibition is thus crucial to understanding the reactions and writings of visitors. The difficulty of conveying emotional experience also explains why contemporaries considered poetry to be better than prose for expressing and communicating visitors’ experiences.” 6
I curated a Content Set in Gale Digital Scholar Lab comprising a range of documents contemporary to the 1851 Great Exhibition, to see if I could determine the prevalent emotions associated with the event using distant reading methodologies. The documents included newspaper content, monographs, letters, reports, and essays. An initial Ngrams analysis helped identify recurrent OCR errors and irrelevant words, which I was then able to remove using a targeted Cleaning Configuration.
Initial analysis highlighted the range of raw materials discussed across the Content Set. Parsing the CSV exported list of unigrams, it became apparent that the top words were primarily focused on the raw materials and manufacturing methods of the items on display, rather than sentiment associated with a visit to the event.
To confirm this finding, I ran a Topic Modelling analysis, choosing to return 50 top topics with 10 words per topic. Again, none of these topics focused on visitors’ emotional connections with the Exhibition. This was an unexpected result given the large number of topics, and implies perhaps that reporting was focused on facts and figures, rather than first person accounts of the event.
The final tool run was Sentiment Analysis itself. The results were concentrated in the neutral/positive regions of the Overall Content Set Sentiment analysis output. The visualization in Gale Digital Scholar Lab is interactive, and hovering over each point on the analysis enables a researcher to expand the details of individual documents to examine sentiment score output across the text, and then to click into the document itself to ‘close read’ the material. This methodology proved most productive in developing an understanding of the nuances of emotion in the Content Set. While the results of the initial tool runs didn’t immediately return words traditionally associated with positive emotion, the combination of close examination of tool output and reading of individual texts helped substantiate and refine initial results.
Enabling More Granular Analysis
The update to Gale Digital Scholar Lab’s Sentiment Analysis lexicon provides options to generate more granular analysis results, since the tool scores a wider range of words than before. The experiment to track sentiment towards the Great Exhibition of 1851 highlights the value and importance of critical tool use – switching between curation, cleaning, examining results by ‘close reading’, and then continuing to refine the Content Set and tool setup. Sentiment Analysis also works well when used in conjunction with the suite of tools in the Lab.
Approaching analysis from multiple angles helps the researcher become familiar with the data, potentially laying the groundwork for new interpretations or conclusions. The results of ‘distant reading’ the Great Exhibition material were not as clear cut as the conclusions reached in Cantor’s article, but the scope of the dataset was broader and certainly trending towards positive sentiment. Further curation would prove useful here to refine the results, perhaps incorporating personal accounts while excluding some of the advertisements and reports.
If you enjoyed reading about the updates to the Sentiment Analysis tool in Gale Digital Scholar Lab, you might like to check out the webinar that blog post author Sarah Ketchley recorded which provides more detail about this fascinating tool. >>View Webinar
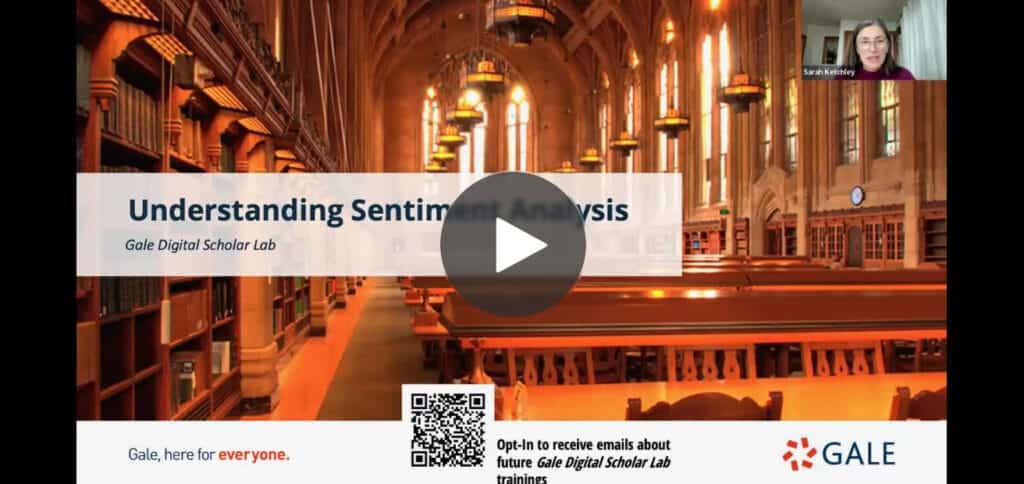
You might also like the other blog posts in our ‘Notes from our DH Correspondent’ series, which include:
- Groups and Notebooks: Using Gale Digital Scholar Lab’s latest features in the DH classroom
- Digging into Datasets in Gale Digital Scholar Lab
- Birds of a Feather, Work Together – Gale Digital Scholar Lab: Groups
- King Tut and Digital Humanities: A Pedagogical Case Study
- Working with Datasets, A Primer
- A Global Community: Learning and Networking Opportunities for Digital Humanists
- Zoë Wilkinson Saldaña (2018) “Sentiment analysis for exploratory data analysis”, Programming Historian, https://doi.org/10.46430/phen0079
- Finn Årup Nielsen (2011) “A new ANEW: Evaluation of a word list for sentiment analysis in microblogs”, Proceedings of the ESWC2011 Workshop on ‘Making Sense of Microposts’: Big things come in small packages, 93-98 https://doi.org/10.48550/arXiv.1103.2903
- James A. Russell (1980) “A circumplex model of affect”, Journal of Personality and Social Psychology, 39(6), 1161 and James A. Russell (2003) “Core affect and the psychological construction of emotion”, Psychological Review, 110(1), 145.
- For context and a brief history of the Great Exhibition, see: Ed King (2007) “The Crystal Palace and Great Exhibition of 1851”, British Library Newspapers, Gale, https://www.gale.com/intl/essays/ed-king-crystal-palace-great-exhibition-1851
- Geoffrey Cantor (2015) “Emotional Reactions to the Great Exhibition of 1851”, Journal of Victorian Culture, 20:2, 230-245, https://doi.org/10.1080/13555502.2015.1023686
- Ibid., p. 244, https://doi.org/10.1080/13555502.2015.1023686