│By Dr. Sarah L. Ketchley, Senior Digital Humanities Specialist, Gale│
This month’s blog post will discuss datasets – what they are, and how they might be used by a researcher or student who plans to use digital tools to generate answers to questions they have about their data. The timing of this post coincides with the release of Gale Digital Scholar Lab’s newest feature: downloadable datasets, pre-curated for use in the classroom or by individual users. We’ll look at some of the options for working with these datasets, and end with some suggestions for sourcing open plain text data for curation and analysis.
Humanities Text Data
Before discussing the nature of datasets, it’s important to note that we’ll be focusing in this blog post on plain text data which has been generated from the optical character recognition (OCR) output created by scanning archival material. This text data is readily accessible in Gale Digital Scholar Lab alongside the image of the primary source for comparative purposes. Looking at this side-by-side view can help identify recurrent OCR errors that may need cleaning during the data curation process. This is one valuable and unique feature of the datasets in the Gale Digital Scholar Lab.
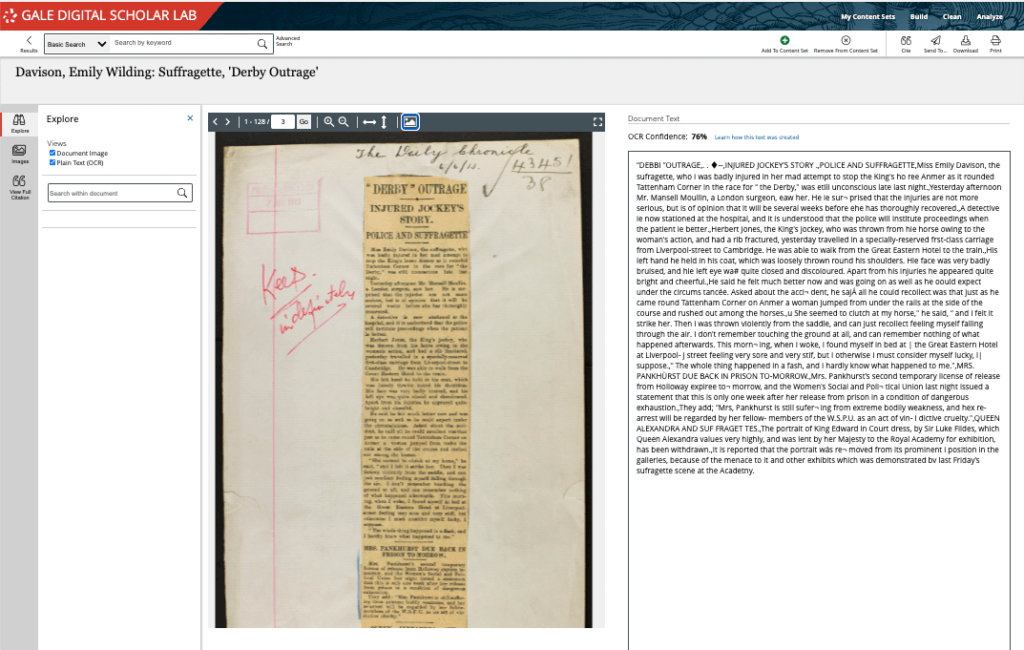
What is a Dataset?
At the most basic level, a text-based dataset is a collection of documents that are related by a common theme, topic or specific event. They may also be documents grouped by a particular type or from a single publication, such as a collection of newspaper articles from The Times of London, or newspaper editorials, personal letters, monographs, novels, and so on. The researcher will determine what the grouping will be, and it is important to capture the ‘meta’ information about the dataset and/or each item, including the original source, dates, copyright restrictions and so forth.
Datasets in Gale Digital Scholar Lab currently include documents grouped around the Stonewall Riots, the Watergate Scandal, and the Suffragette movement, with more collections planned. The metadata is captured at a document-by-document level, and at a dataset/content set level, so users can see at a glance the types of documents in the collection, and where they came from.
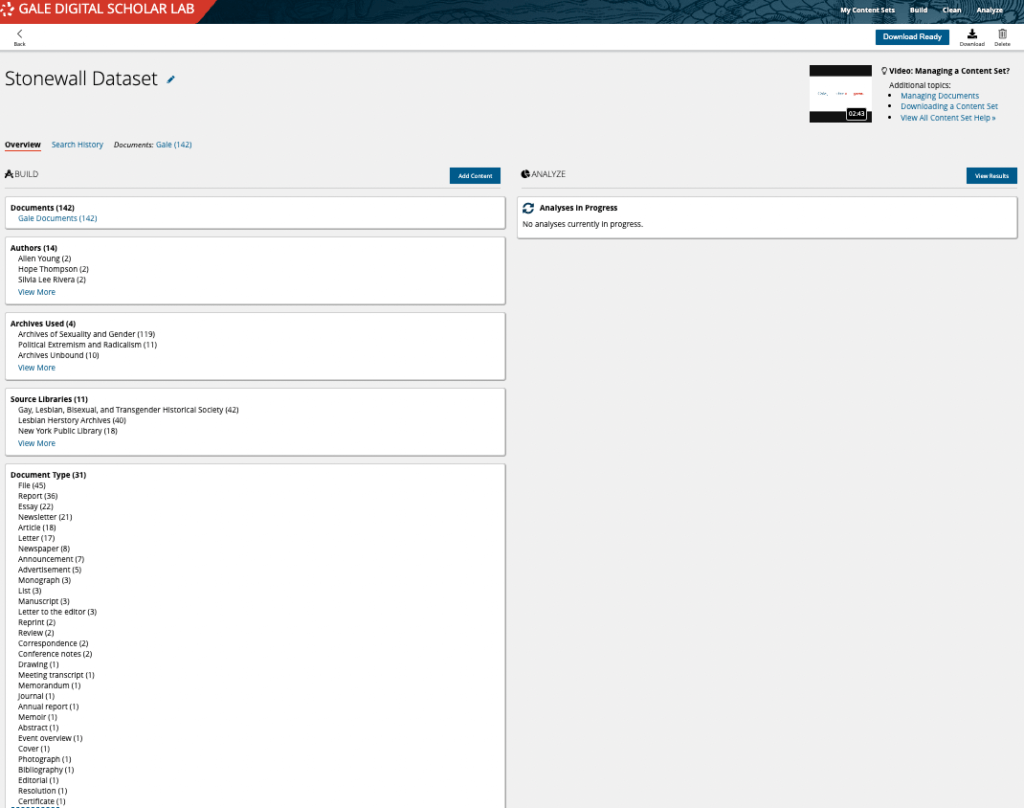
What can you do with a Dataset? Datasets for Text Mining
The type of work a researcher may carry out with the dataset could range from looking for patterns, evidence of reuse over time, most common terms or phrases, recognition of named entitles in the text, such as people’s names, place names and so on. This type of work is broadly named “text mining.”
“Text mining is a research practice that involves using computational analysis to discover information from vast quantities of digital, free-form, natural language, unstructured text.”
(Definition modified from UT Arlington “Text Analysis” Research Guide)
In this definition, computational analysis is the use of computer algorithms to analyse texts. There are several different analysis methods you can employ to accomplish this analysis, to achieve the research goals detailed above. These methods range from quantitative analysis including simple word frequencies, or ngrams, to qualitative analysis such as sentiment analysis, clustering, or building topic models.
How much data (or how many texts) do you need when undertaking a text mining project? The datasets we provide in Gale Digital Scholar Lab will run to around 200 documents. This is an ideal number to begin with: not too large to cause headaches as you begin wrangling data, but not too small that the analysis results you generate are without merit. Of course, as you become more comfortable with the process of working with data, the quantity of material you curate and analyse could grow. There’s not an exact threshold you need to meet, but generally the more data you compile, the more meaningful your results.
The final section of the definition references digital, free-form, natural language, unstructured texts. The key term here is unstructured. When creating visualisations, we can break down the type of data into two formats: structured and unstructured. Unstructured texts are data not formatted according to an encoding structure like HTML or XML, whereas structured data is generally in the form of a spreadsheet or has been encoded using a lexicon. It’s worth noting that when text-mining unstructured data, the computational analysis often results in the creation of structured results through the process of creating statistical/tabular data.
Datasets in Gale Digital Scholar Lab
There are a couple of ways to access pre-curated datasets in Gale Digital Scholar Lab. The first is to navigate to the ‘Learning Center’ then choose ‘Datasets’ from the sidebar menu. Here you will find detailed contextual information about the theory and practicalities of accessing the material, along with summary metadata for each of the datasets. You can then click ‘Get a Copy’ which will add the selected dataset to your ‘My Content Sets’ area.
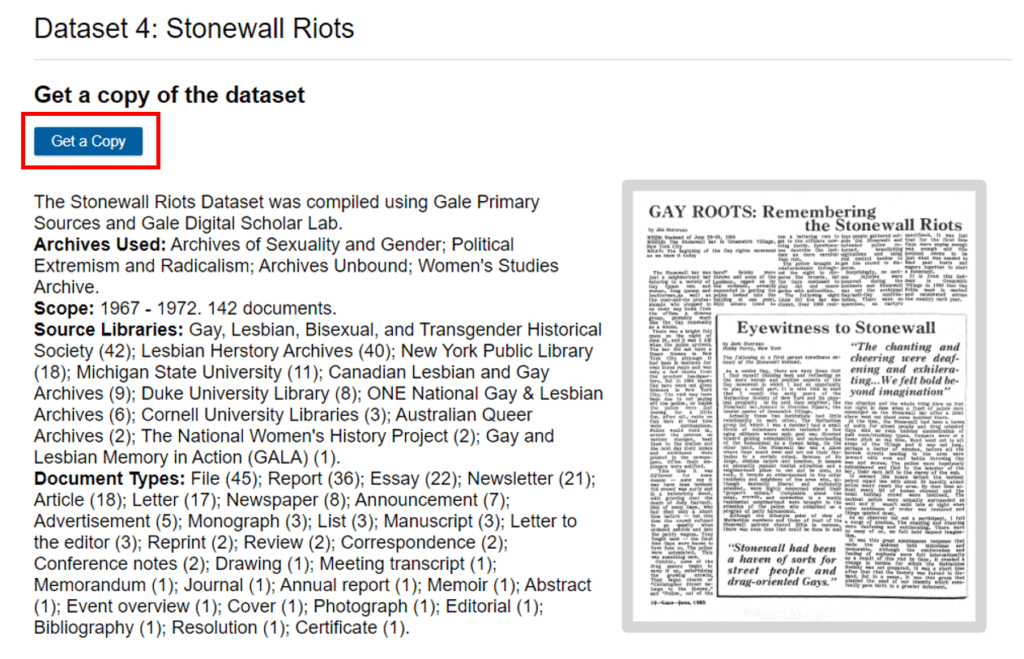
Another way to access datasets is via the Sample Projects. The Datasets differ from the Sample Projects in that they simply provide you with a collection of raw primary source data that you are free to use as you wish. However, the three Sample Projects can also function as raw datasets if the topics interest you, and you want to work with the data further without the Sample Project guidelines. If you click ‘Get a Copy’ the entire content set will be copied to your ‘My Content Sets’ area.
Next Steps
Once your selected dataset is copied into ‘My Content Sets’, you have a few choices you can make about how to work with the data.
- If you know you want to take the material out of the Gale Digital Scholar Lab, you can simply click the ‘Download’ button and a .zip folder will download to your computer. Note that you have some cleaning options prior to download. You can choose the Lab’s default clean, any clean configurations you’ve already created, or no clean at all.
- If you choose ‘no clean’, you have the option of working with the text outside of the Lab to manually clean data, or use another tool or method for the purpose.
- You can analyse the downloaded dataset with external analysis tools.
- You can continue working with the dataset in the Lab – cleaning it, and using any or all of the six analysis tools to ask questions of your data.
- If you do export the plain text dataset to work with outside of the Lab, you can re-import it using the ‘Upload’ function, provided it is still in plain text format.
More Datasets
We’ll be adding to the menu of datasets in the coming months. These datasets will be handpicked from the extensive collections in Gale Primary Sources. In the meantime, a few recommendations for open source datasets and data repositories include:
- Internet Archive
- Project Gutenberg
- Google Books
- Hathi Trust
- JSTOR Data for Research
- PubMed Open Access Subset
- Open American National Corpus
- Kaggle
- English-corpora.org (BYU)
- Data is Plural (Jeremy Singer-Vine)
- DH Toychest (Alan Liu)
Remember to ask your librarian what material is available for text mining at your institution too.
You can import datasets of plain text documents – up to 10MB at one time – into Gale Digital Scholar Lab for analysis, where they can be used as standalone collections, or intermingled with Gale Primary Sources archives, based on your institution’s holdings. This highlights the extensibility of Gale Digital Scholar Lab, which is particularly valuable for classroom use across the humanities and social sciences.
In Summary
Datasets provide the raw material for analysis using DH methodologies. The grouping has been described as a ‘bag of words’ that a computer algorithm then sorts through to categorise, count, group, or reorder. Starting with a small compilation of thematically connected material is an excellent way to learn about the process of text mining.