│By Sarah L. Ketchley, Senior Digital Humanities Specialist, Gale│
This post explores the iterative process of digital humanities project work in Gale Digital Scholar Lab, which provides a user-friendly interface for text mining historical primary source documents from Gale Primary Sources and plaintext material uploaded by researchers. The post discusses how each stage of the curation and cleaning process (Build, Clean, Analyse) is impacted by the need for a flexible and regenerative mindset and workflow that is less linear in nature, more cyclical and iterative.
For those new to the field of Digital Humanities (DH), the process and workflow of building a DH project can come as a surprise, comprising as it does a series of disparate and non-linear steps that cumulatively combine to create research output. Paige Morgan’s article, “The consequences of framing digital humanities tools as easy to use,” highlights some of the pitfalls of oversimplification of digital tools, platforms and processes. Doing so can alienate users if they feel that they should understand what is being presented as an easy process, yet they are struggling to do so.
Project Building in Gale Digital Scholar Lab
One of the goals in developing Gale Digital Scholar Lab was to create a platform to meet the needs of a variety of users, including those who are new to text mining, and those who have a measure of experience, without undermining the nuances of process in preparing Optical Character Recognition (OCR) texts for quantitative and/or qualitative analysis. A primary aim is to provide context at each stage of data collection, curation, and analysis. In the platform, this workflow is described as “Build”, “Clean” and “Analyse”. Here we’ll consider what this looks like in practice, since it’s not strictly a linear workflow. It is better described as “iterative”, which can be confusing to those new to DH research and project work. The concept of “iteration” has been explored as a keyword definition in Digital Pedagogy in the Humanities, which highlights the repetitive, cyclical nature of the process of problem-solving and improving outcomes as a stage in the labour of “doing digital humanities” whether as a researcher, an educator or as a student.
The approach a researcher takes in engaging with the text data can have an impact on the nature and amount of iterative work that needs to be completed. Does the researcher have a set question, or not? Choosing a tool that leverages unsupervised learning techniques, such as topic modelling for example, will find patterns in a group of texts that may not be immediately apparent. This tool is often used when the researcher doesn’t have a specific question, or perhaps wants to get a sense of the thematic content of a collected data set.
Searching as an Iterative Process
We have all put keywords or search terms into a search engine like Google or Duck Duck Go to see which results are returned. Sometimes these are what we were hoping for, sometimes not. If they aren’t satisfactory, we can tweak our search terms and try again. Searching in Gale Digital Scholar Lab follows a similar pattern. A researcher may have a good idea of what they’re looking for, and the search terms they intend to use, such as author name, publication date, publication and so on. But often the list of search results returned can be too extensive to usefully work with and will need to be trimmed down. At this point the researcher will iterate on their search, further refining and filtering the terms using some of the options available. It may be that a brief review of individual documents reveals that some can be cut from the content set because the OCR confidence is not high enough. Or perhaps advertisements are not relevant to the research and can usefully be removed using the inbuilt filter for “Document Type”.
In this process of building a content set, adding and removing documents takes place before, during and after running analyses. Searching is certainly the starting point of any project (unless documents are added using the “Upload” feature in the platform), but it should be viewed as a flexible, evolving and iterative process.
The Process of Cleaning OCR Text
Moving on to the “Clean” phase, the researcher will make choices about which elements in the OCR text should be excluded, replaced or kept. This involves some close reading of the original document and its OCR output but may also include a preliminary run of analysis tools like Ngrams to identify the most prevalent terms in the content set, and whether they should be excluded from further investigation. Running a unigram analysis on an uncleaned content set will return this information (words or phrases to be excluded); downloading a .csv file of the results, then pasting the irrelevant material into the stop word list in the “Clean” tool will immediately remove these from the analysis.
The updated Clean configuration can then be tested on a small subset of 10 documents, downloaded by the researcher in a .zip file which also contains the same 10 uncleaned documents. A side-by-side comparison of this material will help identify what has slipped through the cleaning net, and the configurations that may need to be tweaked.
The researcher will then return to Clean and make further choices. The length of time this process takes is significant and any project workflow will almost inevitably involve multiple iterations of this content evaluation. There are two ways to keep track of choices made during each run of the Clean tool:
1. By creating an informative title for the configuration.
2. By keeping complete “Configuration Notes” to document process and decisions.
Iteration and Analysis
There are six tools for text-mining analysis in Gale Digital Scholar Lab.
Three of these tools are quantitative (Ngrams, Parts of Speech and Named Entity Recognition), and three are qualitative (Topic Modelling, Clustering and Sentiment Analysis). The first group tend to produce results which are static and predictable in nature – they are raw counts of data in a user’s content set.
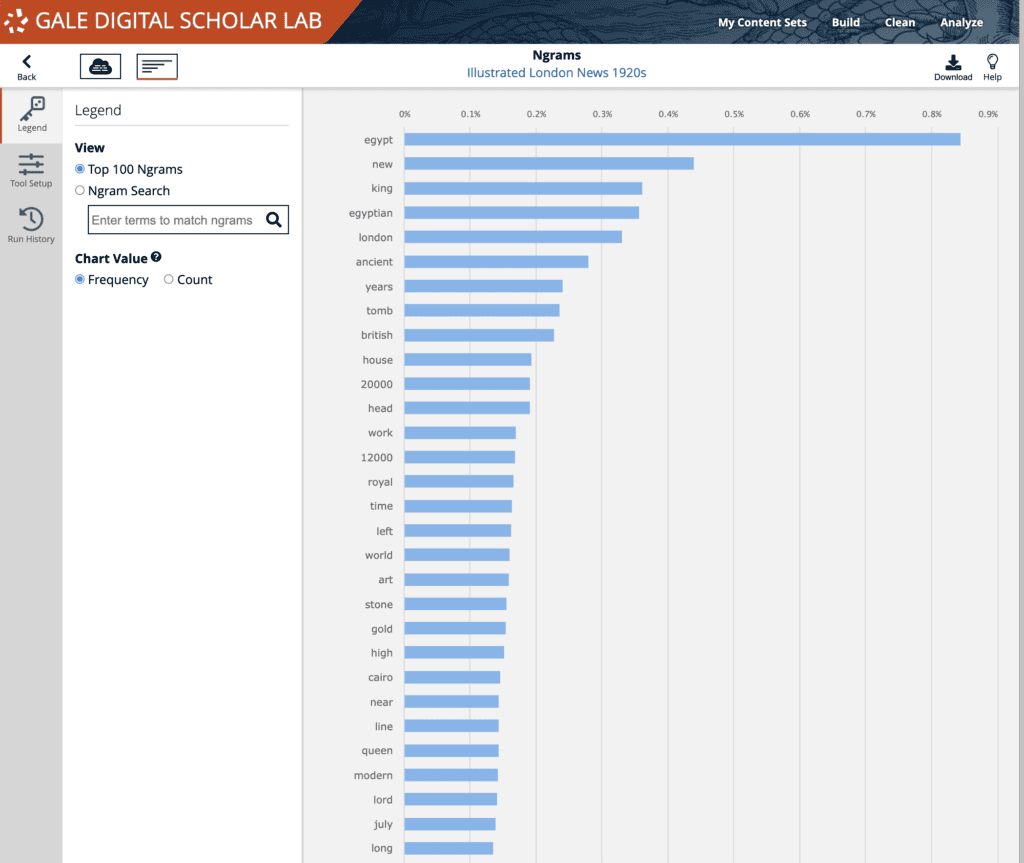
Analysis results from the second group require a little more input from the scholar. Topic modelling, for example, won’t give exactly the same results each time the tool is run. And the user must determine what any given theme should be named – Topic 2 may contain the words “horse, rider, stable, gallop, saddle, pasture, jumps”, so the researcher might decide to rename the topic to something like “Equestrian Pursuits”. In all cases, examining the visualisation output can highlight outlying documents that should be removed to make the visualisation more compelling. If this is the case, the analysis will be re-run, and the results examined again. Perhaps there are too many documents with OCR errors in the results, in which case the researcher would either return to “Search” to filter them out using the OCR Confidence filter, or they could use the Clean tool to prepare the documents for analysis. This back and forth will continue until analysis results and visualisations are satisfactory.
Iteration is key
The interplay between each of the stages of work in Gale Digital Scholar Lab provides an opportunity for the researcher to revisit and revise documents, configurations and analysis outputs, offering considerable fine-grained control over the quality of the final output from the platform. The process involves making a myriad of small decisions, and iterating on the composition of content sets and the configuration of tools until a satisfactory – and perhaps unexpected – outcome is achieved.
If you enjoyed reading about the iterative processes involved in Digital Humanities scholarship, and using Gale Digital Scholar Lab, you may like to read more posts in the Digital Humanities category on this blog, including:
- New Experience for Gale Digital Scholar Lab
- New Learning Center added to the Gale Digital Scholar Lab
- Students at the University of Helsinki use the Gale Digital Scholar Lab
- Lifting the lid on how we created the Gale Digital Scholar Lab
- How the Gale Digital Scholar Lab made digital humanities less daunting
- Using the Gale Digital Scholar Lab in the Classroom